Cardiovascular diseases (CVDs) are the leading cause of death globally, representing a significant concern for public health. Coronary artery disease (CAD) accounts for nearly half of all CVD deaths worldwide.1,2 Despite improvements in primary prevention and treatment, and the development of several risk stratification algorithms to predict 10-year cardiovascular mortality or lifetime risk in different populations, the prevalence of CAD continues to rise.1,3,4 Thus, the identification of novel biomarkers for early and accurate recognition of at-risk individuals is of paramount importance.
Circulating microRNAs (miRNAs) represent optimal non-invasive potential candidates for both diagnostic and prognostic purposes.5 Due to their high stability, phylogenetic conservation and resistance to degradative enzymes, and due to their easy detectability in body fluids thanks to advances in molecular detection techniques, miRNAs may serve as stable and reliable reporters of disease onset and progression. MiRNAs are non-coding RNAs that play a role as ‘fine-tuners’ of gene expression.6 They are key regulators of the cardiovascular system, including heart muscle contraction and growth, conductance of electrical signals, vessel wall homeostasis, response to vascular injury and tissue repair.7 Dysregulation of their expression is involved in cardiovascular pathophysiology, and circulating miRNAs may contribute to the interaction between inflammatory and vascular cells, systemic inflammation, and oxidative stress, and thus to CAD pathogenesis (Figure 1).8–10
In the past two decades, great attention has been paid to the role of miRNAs as diagnostic and prognostic biomarkers, as well as therapeutic targets in heart disease. In the present review, we summarise existing knowledge on miRNAs as biomarkers for risk stratification and prognosis in CAD.
MicroRNA Function and Biogenesis
MiRNAs are short (22–25 nucleotides), non-coding, single-stranded RNA molecules that regulate the expression of 60% of protein-coding genes at the post-transcriptional level by complementary binding to the 3´ untranslated region of the target mRNA, leading to inhibition of translation or mRNA degradation.11,12 They could also interact with the 5´ untranslated region, causing gene expression silencing (Figure 2).13
MiRNA canonical biogenesis consists of several steps: transcription, processing, splicing, export to the cytoplasm, maturation and target binding.14 Briefly, a pri-miRNA (a large structure composed of sequences for several miRNAs) is synthesised by the RNA polymerase II enzyme in the nucleus. Pri-miRNAs are cleaved by the RNA-specific RNase-III-type endonuclease, Drosha, with its cofactor DGCR8, to produce pre-miRNAs (70 nucleotides), which are exported in the cytoplasm via exportin-5 and RanGTP-binding protein. Pre-miRNAs are further processed by Dicer (the cytoplasmic RNase-III endonuclease) into a double-stranded miRNA duplex and loaded on the RNA-induced silencing complex, where the guide and passenger strands are selected by Argonaute protein.15 The RNA-induced silencing complex leads the guide strand to bind to its target mRNA through a complementary interaction between the miRNA and target mRNA seeding sequences.14
Extracellular miRNAs are secreted into the blood circulation through several carriers to protect them from digestion: packed in exosomes, microparticles or lipid vesicles; bound to Argonaute-2 or nucleophosmin-1 proteins; or linked with high- or low-density lipoproteins.16–18
MicroRNAs as Circulating Biomarkers
Extracellular miRNAs have been detected in different body fluids, such as pleural, peritoneal, cerebrospinal, seminal and amniotic fluids, urine, breast milk, colostrum, saliva, tears and blood.19 Differently from intracellular mRNAs, extracellular miRNAs circulating in the blood show great stability under harsh conditions (such as extreme environmental basic pH, high temperature, multiple repeated freeze–thaw cycles or prolonged storage) and resistance to endogenous RNase activity.20 Moreover, miRNAs are evolutionarily conserved among species, allowing translation from preclinical models to clinical practice.21 Finally, they are easily detectable by sensitive techniques, such as reverse transcription quantitative real-time polymerase chain reaction (RT-qPCR).
These properties raised enormous interest in their use as biomarkers for various diseases. Indeed, since 2008, when Lawrie et al. first demonstrated the potential of serum miRNAs as biomarkers for diffuse large B-cell lymphoma, several studies have identified specific miRNA expression signatures in cells, tissues or biological fluids, demonstrating their association with human diseases and/or their prognosis.22 Although the vast majority did not prove to be clinically relevant, specific miRNA expression signatures, especially in serum or plasma, have shown promise as minimally invasive tools for diagnostic/prognostic purposes. The potential use of circulating miRNAs for diagnostic purposes has been extensively explored in CAD.23,24
Circulating MicroRNAs in Coronary Artery Disease Risk Assessment
Several risk algorithms have been developed for CVD primary prevention, mostly based on traditional cardiovascular risk factors (TRFs; e.g. sex, age, smoking habit, total and HDL cholesterol, systolic blood pressure, treatment, diabetes).3 However, although they represent very useful tools to help clinicians stratify patients based on risk and guide treatment options, the performance of these models is suboptimal, showing the need for new refined and accurate risk stratification models, including novel biomarkers.25 Yet, studies on the role of circulating miRNAs in cardiovascular risk stratification are rather sparse.
Primary Prevention Settings
The main results of studies assessing circulating miRNAs potential as predictors of future cardiovascular events in the general population (i.e. in primary prevention) are shown in Table 1, along with sample type, detection and normalisation methods, miRNA regulation, primary outcome, and factors and covariates for adjustment in multivariable analyses.
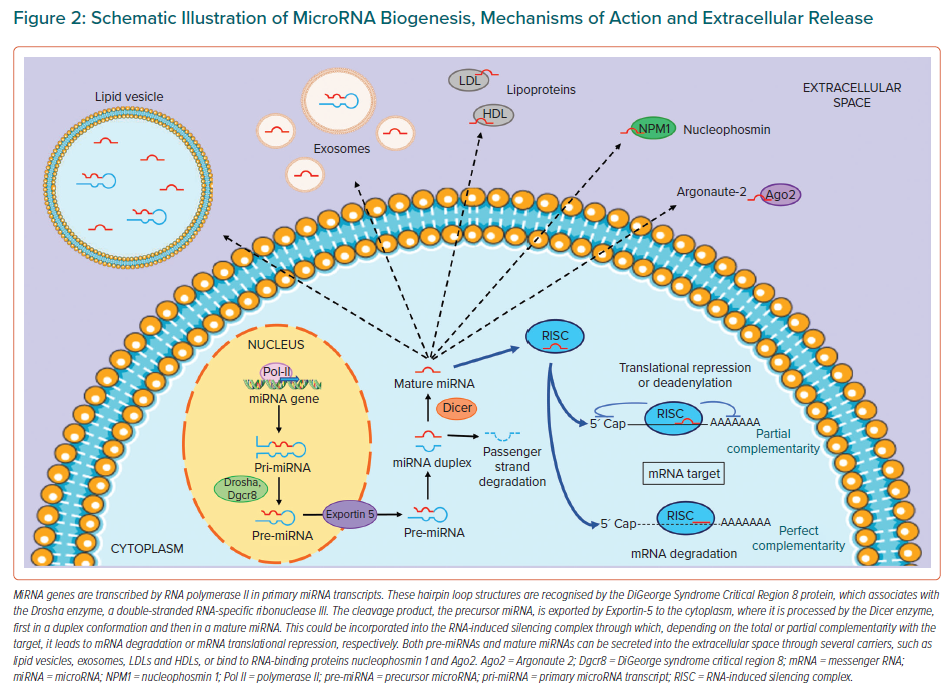
An early study that prospectively sought an association between basal miRNA levels and incident MI was that of Zampetaki et al., reporting in 2012 a significant relationship between plasma levels of three miRNAs and MI (miR-126-3p positively associated, while miR-197 and miR-223 inversely associated).26 Using RT-qPCR, they evaluated the baseline plasma expression of seven miRNA candidates (miR-24, miR-126, miR-140, miR-150, miR-197, miR-223 and miR-486) in a population-based cohort of 820 participants (aged 40–79 years) in the Bruneck Study.27 A total of 47 participants experienced fatal or non-fatal MI within the 10-year follow-up period. Seven miRNAs emerged as promising from an initial screening using Taqman miRNA arrays, covering 754 small non-coding RNA, on eight pooled samples of participants with or without atherosclerotic vascular disease matched for various TRFs. They used two distinct approaches to select the subset of miRNAs with the highest predictive ability for future MI (i.e. stepwise Cox regression analyses followed by comparison of the emerging models’ Akaike information criterion; L1 penalisation, based on least absolute shrinkage and selection operator algorithm), which identify the same miRNA signature.
Then, risk estimates for the three miRNAs selected were computed by standard Cox regression analysis adjusting for a set of TRFs. They showed that adding the three miRNAs to a Framingham Risk Score (FRS) model for hard endpoints of coronary heart disease slightly increased both the C-index, the net reclassification index, and the integrated discrimination improvement.28
Finally, to determine the cellular origin of these three miRNAs, they performed a miRNA screening in preparations of thrombin-activated platelets, and an interventional study by subjecting 11 healthy volunteers to limb ischaemia-reperfusion (which induces platelet activation), assessing plasma levels of 30 candidate miRNAs at various time-points. Through computational analysis, they identified a temporal cluster containing all three MI-predictive miRNAs, characterised by early and sustained elevation, and consisting of miRNAs predominantly expressed in platelets.
In 2016, Bye et al. described a signature of five miRNAs (miR-106a-5p, miR-424-5p, let-7g-5p, miR-144-3p and miR-660-5p) that could predict future fatal acute MI (AMI) in healthy individuals.29 They assessed by RT-qPCR the baseline serum levels of a panel of 179 miRNAs in a prospective nested case–control study cohort of 112 healthy individuals (aged 40–70 years) participating in the HUNT study with a 10-year observation period.30 This derivation cohort consisted of 56 cases suffering from fatal AMI within 10 years and 56 controls remaining healthy matched for risk factors. MiRNAs found to be differentially expressed in the screening were then analysed in an independent validation cohort of 100 healthy participants from the same study, with 50 suffering from fatal AMI and 50 risk factor-matched controls reporting no cardiovascular events: 10 miRNAs were confirmed to be differentially expressed in cases versus controls.
The exclusion criteria for both cohorts included previous cardiovascular events and several comorbidities. Interestingly, a sex-specific association was observed, as miR-424-5p and miR-26a-5p were exclusively related to risk in men and women, respectively.
Finally, a model consisting of the five miRNAs with the highest predictive ability for future AMI in both sexes was identified by conditional logistic regression followed by a comparison of the Akaike information criterion of the models, providing 77.6% overall correct classification. Adding the five miRNAs to the FRS significantly increased the receiver operating characteristic area under the curve (AUC).
Three years later (Velle-Forbord et al.), leveraging the population-based HUNT cohort, the same group showed that a combination of another five miRNAs (miR-21-5p, miR-26a-5p, mir-29c-3p, miR-144-3p and miR-151a-5p) added to the FRS was the best predictive risk model for both fatal and non-fatal MI.31 They performed a case–control study, testing by RT-qPCR in baseline serum samples of 195 participants (aged 60–79 years) the 10 candidate miRNAs (let-7g-5p, miR-21-5p, miR-26a-5p, miR-29c-3p, miR-106a-5p, miR-144-3p, miR-151a-5p, miR-191-5p, miR-424-5p and miR-451a) previously explored for their potential to predict future fatal MI.29
Specifically, during the 10-year follow-up, 36 and 60 participants experienced either a fatal or a non-fatal MI, respectively, whereas 99 age- and sex-matched controls remained healthy. They used the same logistic regression approach for the best subset to identify the miRNA signature that, along with the FRS for hard CAD, most effectively predicted future MI. The model was evaluated by 10-fold cross-validation for the AUC, and the miRNA panel was shown to add predictive value to the FRS, leading to a significant increase in the AUC. Of note, the addition of other important CAD risk factors (waist:hip ratio, triglycerides, glucose, creatinine) to the FRS did not significantly improve risk prediction.
In 2017, Keller et al. tested the ability of another panel of five candidate miRNAs in predicting cardiovascular outcome.32 Selected miRNAs included miR-34a for its role in cardiac ageing, miR-223 as a regulator of inflammation, and the cardiac-enriched miR-378, miR-133 and miR-499 associated with either cardiac hypertrophy or myocardial damage.33–37 They used as the derivation cohort a sample of low-to-intermediate-risk primary care patients (178 German healthy individuals, median age 55.5 years, interquartile range: 46–69 years) randomly chosen from the prospective longitudinal DETECT study, and as the independent validation cohort, a general population sample (129 healthy participants, mean age 74.7 ± 8.2 years) from the SHIP.38,39
Endpoints were overall mortality and/or cardiovascular events (cardiovascular death, non-fatal MI or need for coronary revascularisation). Twenty-one participants from the DETECT study reached the combined endpoint within a 5-year follow-up period, 12 of whom died. Baseline plasma levels of the five selected miRNAs were assessed by RT-qPCR. Cox proportional hazards models, adjusted for age, sex and FRS, showed that 5-year all-cause mortality was associated with reduced miR-133 levels and that there was a trend for the association with lower miR-223 levels. Importantly, a score from the multivariate miRNA panel, calculated by a logistic regression model, showed a robust association with overall mortality, withstanding adjustment for the same covariates. No significant association with the combined outcome was observed for either individual miRNAs or the entire panel. However, the 5-miRNA panel improved the ability of the FRS to identify patients at risk to die within 5 years, as indexed by a significant increase in AUC.
Then, the authors explored the use of the miRNA panel in primary prevention: 64 individuals without a CVD history, who died within a 12-year follow-up, were randomly chosen from the SHIP study, and age- and sex-matched 1:1 with 65 controls. The 5-miRNA panel confirmed in this validation cohort its ability to predict all-cause mortality, independently of established risk scores, such as the FRS or SCORE.28,40 The addition of the 5-miRNA panel significantly improved risk stratification models based on the FRS or the SCORE in predicting mortality, increasing both net reclassification index and integrated discrimination improvement.
Using a different approach, in 2020, Gigante et al. tried to identify circulating miRNA signatures that predict major adverse cardiovascular events (MACE; defined as sudden cardiac death, first-time MI or angina requiring hospitalisation) in middle-aged men and women.41 To this end, they took advantage of a prospective Swedish cohort of 60-year-old men and women from the Stockholm Study, selecting the first 100 MACE-presenting individuals and 100 MACE-free individuals in an 11-year follow-up (matched for sex and time of inclusion in the cohort).42
They initially screened a panel of 754 miRNAs, using TaqMan OpenArrays, in baseline plasma samples. Using a random effects logistic regression, adjusted for common TRFs, they identified nine miRNAs potentially associated with an increased rate of future MACEs, being miR-145-3p associated with the largest estimated risk increase, and miR-720 associated with reduced MACE risk.
Then, they identified 16 interacting miRNA pairs associated with an increased probability of an event, by random effects logistic regression models that included only two miRNAs and their interaction (without covariates). Notably, miR-320b was present in all interacting miRNA pairs associated with the risk of MACE, and the expression level of miR-320b influenced the strength of the association of the paired miRNA (the higher miR-320b expression level, the greater the risk associated with each miRNA).
Finally, they performed target prediction of miR-320b and the 16 identified interacting miRNAs, and grouped miRNAs in four clusters: three of these clusters (cluster 1: miR-320b plus miR-145-3p, miR-128a, miR-548d-3p; cluster 2: miR-320b plus let-7g-5p, let-7d-5p, let-7e-5p, miR-196b-5p, miR-191-5p, miR-324-3p; and cluster 4: miR-320b plus miR-301b,miR-340-3p, miR-376a) were linked with cardiovascular development and function, and CVDs, as documented by enrichment pathway analysis of the targets. They then sought validation using an external prospective cohort (58 patients with incident MI and 60 sex-matched controls within 1 year from initial serum sampling, mean age 60 ± 5 years) from the HUNT study.30 Although not significant, they observed a similar pattern of association with the risk of MI, and a trend in a progressive increase in MI risk estimates for miRNAs from clusters 2 and 4.
The same year, Wang et al. reported a negative correlation of miR-423-3p with the risk of CAD events, showing added predictive ability to TRFs.43 To identify the most specific miRNAs associated with CAD, they implemented a multistep design. They initially performed a screening by miRNA sequencing on pooled samples of serum or peripheral blood monocytes from 10 CAD patients and 10 healthy controls, as well as hypoxia-treated vascular endothelial cells (ECs). Out of the 1,022 miRNAs detected in patient serum and 976 in controls, they selected 48 candidates for detection in serum, which were also dysregulated in CAD monocytes and hypoxic ECs. By PCR product sequencing, the authors found that five miRNAs (miR-10a-5p, miR-126-3p, miR-210-3p, miR-423-3p and miR-92a-3p) were specifically detectable in serum.
Finally, the serum levels of these five miRNAs were measured by RT-qPCR in two cohorts of sex-matched CAD patients and controls (n=39 versus n=39 and n=30 versus n=21, respectively): miR-10a-5p and miR-423-3p emerged as the best-associated candidates, consistently showing a significantly lower expression in CAD patients than in controls. Therefore, the authors used Cox regression analysis to assess the ability of these two miRNAs to predict CAD events in a large-scale general population of 2,812 individuals (China-CVD cohort, mean age 51.2 ± 7.9 years), with a median follow-up of 6 years. CAD events (n=64) were non-fatal, and included AMI and subsequent MI. After adjustment for age, sex and TRFs, they found that only miR-423-3p was associated with CAD event risk. The addition of miR-423-3p to TRF-based models improved prediction performance, including AUC and net reclassification index.
Challenges of MicroRNAs as Predictive Biomarkers
The potential of circulating miRNAs as non-invasive biomarkers for risk stratification is an attractive field. However, miRNAs detected so far have shown poor reproducibility between studies (Table 1). Specifically, miR-233 was shown to be predictive of MI or all-cause mortality in two different studies.26,32 Surprisingly, miR-144-3p was part of two different panels reported as predictive of MI by the same group in two subsequent studies of samples from the HUNT cohort, but in the first its levels were increased in cases, in the second its levels were decreased.29,31 Similarly, let-7g-5p had decreased levels in cases with future fatal MI in the study by Bye et al., and instead increased in patients who developed MACE in the study by Gigante et al.29,41
The conflicting results reported above could be due to several factors. Sampling is a critical issue; specifically, the choice of anticoagulant and blood fraction could affect the quality of results. Indeed, variability in miRNA detection levels has been observed between serum and plasma.44 An utmost critical step affecting result reproducibility is the normalisation method used. Normalisation strategies include the use of endogenous reference miRNAs, spiking-in of exogenous miRNAs or global mean normalisation to account for technical variability.
Although miR-16-5p and small nucleolar RNA U6 have commonly been used in several studies, there is a substantial lack of consensus on endogenous miRNAs.45 Their amount in plasma or serum samples may be affected by several factors, including haemolysis, physical activity and fasting, and these miRNAs have been shown to be invariant only in specific situations.46 Conversely, a major drawback of the spike-in method is that it is unreliable when quantification of the extracted RNA is not possible, which is often the case for circulating miRNAs. In fact, equal volumes of serum/plasma may contain variable amounts of miRNAs.46
Finally, mean/median normalisation methods are another possibility for quantifying miRNA expression, but produce data that are difficult to compare with other normalisation methods. It is clear that different normalisation methods, as those used in the above-mentioned studies, profoundly affect the final results.
Another issue of fundamental importance is that the clinical outcome was often different among primary prevention studies, ranging from MACE to fatal or non-fatal MI to death from all causes. Clearly, different miRNAs can be expected to predict different events. Furthermore, these studies were conducted on populations of diverse ethnicity, had varying approaches in study design and detection methods, and adopted different statistical models. In addition, there are strong correlations between circulating miRNA levels; thus, different studies may report different, but highly correlated, miRNAs with approximately the same chance of being included in prediction models. Finally, physiological or normal miRNA concentration ranges in blood have yet to be established.
Circulating MicroRNAs and Coronary Artery Disease Prognosis
Risk stratification for future events among patients with CAD is critically important for prognostication. Risk assessment for recurrent cardiovascular events currently relies on myocardial injury markers, such as troponins or N-terminal prohormone of brain natriuretic peptide, but none of them are specific for any particular outcome. This has prompted the search for new reliable biomarkers.
Secondary Prevention Settings
The main findings and characteristics of studies assessing the potential of circulating miRNAs in this context are shown in Table 2, presenting data on acute coronary syndromes (ACS) first and then on chronic coronary syndromes (stable CAD).
In an early study (2011), Widera et al. reported an association of miR-133a and miR-208b levels with the risk of all-cause death in an ACS cohort from Hannover.47 They focused on cardiomyocyte-enriched miRNAs (miR-1, miR-133a, miR-133b, miR208a, miR-208b and miR-499), known to be cardiac selective and rapidly released after AMI.37,48,49 To assess their prognostic value, the authors determined plasma concentrations of the six miRNAs on admission by RT-qPCR in a cohort of 444 consecutive patients (aged 55–73 years) with a final diagnosis of ACS (n=117 unstable angina, n=131 non-ST-segment elevation MI [NSTEMI] and n=196 ST-segment elevation MI [STEMI]). All-cause mortality at 6 months (n=34 patients) was the primary endpoint. They found that miR-133a and miR-208b baseline levels were significantly related to the outcome at the log-rank test, but stepwise Cox regression analyses indicated that they withstood adjustment for age and sex, but not for high-sensitivity troponin T (hsTnT). Moreover, they did not increase the ability of hsTnT in discriminating survivors from non-survivors.
Gidlof et al. used a similar approach to assess the prognostic potential of three cardio-enriched miRNAs (miR-1, miR-208b and miR-499-5p) in patients with suspected ACS, and found that increased baseline plasma levels of miR-208b and miR-499-5p were associated with the risk of death or heart failure (HF) and reduction in systolic function after MI.50 They analysed by RT-qPCR 407 Swedish patients presenting with chest pain suspicious for ACS (n=88 non-MI, n=146 NSTEMI, n=173 STEMI; median age 65 years), after interventional therapies and within 24-72 hours from presentation. A total of 74 patients experienced the combined primary endpoint (death within 30 days of hospitalisation, heart failure, ejection fraction <40%, or cardiogenic shock). Plasma levels of miR-208b and miR-499-5p were associated with the outcome, with significant odds ratios adjusted for age, sex and time from admission to sampling. However, again, they did not withstand adjustment for TnT, and their prognostic accuracy was similar to TnT.
In keeping with this line of research, Olivieri et al. described an association between admission circulating levels of the cardio-miRNA miR-499-5p and 12-month cardiovascular mortality in elderly NSTEMI patients.51 They elected to test the prognostic potential of two miRNAs (miR-499-5p and miR-21) based on previous observations about their diagnostic performance in the same setting.52 They analysed the baseline expression of these miRNAs by RT-qPCR in plasma samples of 142 Italian NSTEMI patients admitted to the coronary care unit with an interval of 4–9 hours from symptoms onset to admission. Cardiovascular mortality at 12 and 24 months was prospectively defined as the primary endpoint. A total of 54 patients (mean age 85.9 ± 5.4 years) died within 12 months, and 88 survived (mean age 81.7 ± 6.0 years). By multivariate analysis using Cox proportional hazards model adjusting for all significantly different variables at admission, they reported a significant increase in the 12-month risk of death among NSTEMI patients whose miR-499-5p admission level was higher than the median. In particular, patients presenting with congestive heart failure had a twofold greater risk of dying within the first 12 months when baseline miR-499-5p exceeded the median. However, the performance of this model was far from optimal, and miR-499-5p was not associated with cardiovascular mortality at 24 months.
Starting with a different hypothesis, Schulte et al. investigated the ability of the three miRNAs described by Zampetaki et al. in a primary prevention setting, to stratify the risk of future cardiovascular events in patients with documented CAD.26,53 Indeed, miR-126, miR-197 and miR-223 were known to be involved in endovascular inflammation and platelet activation, and as biomarkers in the diagnosis of CAD-related conditions.5,23,24 They measured the serum levels of these miRNAs by RT-qPCR in a cohort of 873 German patients with manifest CAD (median age 64 years [interquartile range 57–69 years], selected from the AtheroGene study), of whom 340 had ACS and 533 had stable angina (SA).54 After a median follow-up of 4 years, 18 cardiovascular deaths were recorded, eight among ACS and 10 among SA patients. The authors used ACS-SA stratified Cox regression models, adjusted for age, sex and further TRFs, followed by 10-fold cross-validation of the C-indices, to explore the association with each miRNA, and found that elevated levels of miR-197 and miR-223 were able to predict future cardiovascular death in the overall group. Considering only the ACS subgroup, the prognostic ability of the two miRNAs was even higher, but in SA patients it was not significant. Of note, there was no incremental benefit in prognostic power using any combination of the three miRNAs compared with single miR-197 or miR-223.
The same group (Karakas et al.), followed up on a similar working hypothesis and evaluated the prognostic potential of eight miRNAs they had previously identified as diagnostic of unstable angina.55,56 Using RT-qPCR, they assessed baseline serum concentrations of miR-19a, miR-19b, miR-132, miR-140-3p, miR-142-5p, miR-150, miR-186 and miR-210 in a cohort of 1,112 patients with an angiographically documented CAD (430 with ACS, mean age 63 years, and 682 with SA, mean age 64 years) derived from the above-mentioned study. After a median 4-year follow-up, 78 cardiovascular deaths or non-fatal MI were recorded (43 among ACS and 35 among SA patients).
Specifically, the authors assessed the association of circulating miRNAs with cardiovascular mortality both in the ACS and the overall cohort by Cox regression correcting for multiple testing (controlling the false discovery rate). All measured miRNAs, except miR-142-5p, were likely to predict cardiovascular death in the ACS group, even after adjustment for age, sex plus TRFs, other emerging risk factors or ACS diagnosis (unstable angina, STEMI, NSTEMI). The best predictors were miR-132, miR-140-3p and miR-210, which were able to markedly increase the c-statistics in the ACS cohort. In the overall CAD cohort, five miRNAs (miR-19b, miR-132, miR-140-3p, miR-150 and miR-186) were associated with an increased risk of cardiovascular mortality, again, independent of risk factors and covariates.
In 2014, Jansen et al. used a different approach, and observed that high levels of miR-126 and miR-199a contained in circulating microvesicles (MVs) were associated with a lower rate of MACE in patients with stable CAD.57 In two German cohorts with a total of 181 patients (mean age 66.7 ± 10.2 years) affected by angiographically documented stable CAD, they quantified by RT-qPCR the baseline arterial blood plasma and MV levels of 10 miRNAs involved in the regulation of vascular function and/or expressed by vascular smooth muscle cells or ECs (miR-126, miR-222, miR-let7d, miR-21, miR-20a, miR-27a, miR-92a, miR-17, miR-130 and miR-199a).58 Occurrence of a first MACE, including non-fatal MI, need for revascularisation and death from cardiac causes, was evaluated within a median follow-up period of 6 years, and 55 events were recorded. No association between miRNA plasma levels and MACE was observed. On the contrary, above-median expression of miR-126 and miR-199a in circulating MVs was associated with a reduced risk of MACE, at univariate analysis. Binary logistic regression, adjusting models for TRFs and medication used, showed that the two miRNAs were not associated with baseline characteristics. Importantly, fluorescence-activated cell sorting analysis revealed that ECs and platelets were the main sources of MVs containing miR-126 and miR-199a, respectively.
At variance with the study by Karakas et al., in 2019, Tang et al. reported that plasma miR-142 was a potential marker for MACE prediction in CAD patients undergoing percutaneous coronary intervention on dual antiplatelet therapy with clopidogrel and aspirin.55,59 To select miRNA candidates, the authors first performed a screening by miRNA sequencing of four pooled plasma samples from 115 Chinese CAD patients stratified according to their sensitivity or resistance to aspirin and/or clopidogrel treatment, as documented by platelet aggregation tests. Differential expression analysis between the four subgroups, followed by RT-qPCR validation in plasma pools and 115 individual samples, revealed that six miRNAs (miR-126, miR-130a, miR-142, miR-27a, miR-21 and miR-106a) were positively associated with clopidogrel antiplatelet efficacy. Based on these results, the six miRNAs were tested by RT-qPCR after percutaneous coronary intervention in a prospective cohort of 1,199 Chinese CAD patients (aged 40–80 years). The primary outcome was MACE, including cardiovascular death, MI or stent thrombosis, within a 3-year follow-up period. Multivariable Cox regression analysis, after correction for multiple comparisons by false discovery rate, revealed that a high plasma level of miR-142 was an independent risk factor for MACE, irrespective of age, sex, comorbidities and medications.
Also, quite at odds with several of the abovementioned studies, Mayer et al. reported that low plasma levels of miR-1, miR-19a, miR-126, miR-133a and miR-223 were associated with a significant increase in 5-year all-cause and cardiovascular death in stable chronic cardiovascular patients. 47,50,53,55,60 This study originated as a secondary analysis from the Czech EUROASPIRE Survey data.61 A prospective cohort of 826 patients with chronic vascular disease (mean age 65.2 ± 9.3 years) was enrolled 6–36 months after a vascular event (ACS and/or coronary revascularisation for 487 CAD patients, first-ever ischaemic stroke for the other 339 patients). During a median follow-up of 5.6 years, 167 patients died, including 126 from events considered to be of cardiovascular origin.
Based on previous literature, the authors initially quantified by RT-qPCR the plasmatic levels of 10 miRNA (miR-1, miR-19a, miR-21, miR-34a, miR-126, miR-133a, miR-197, miR-214, miR-223 and miR-499) in a pilot cohort of 100 patients, 50 dead and 50 age- and sex-matched survivors. Baseline levels of the five miRNAs showing statistical differences between the two groups (see above) were then assessed in the full cohort. Using Cox proportional hazards regression, they found an inverse association between each of the five miRNAs, and a significant increase in both all-cause and cardiovascular mortality, despite adjustment for TRFs, primary diagnosis, treatments, increased cardiac troponin I and BNP. Noteworthy, if all five miRNAs were included in a single regression model, only low miR-19a was a significant predictor of both all-cause and cardiovascular mortality, independently of all the other risk factors and covariates. A subgroup analysis showed that low miR-19a predicted all-cause mortality risk only in chronic CAD, but not in post-stroke, patients.
Circulating MicroRNAs and Postoperative AF
Postoperative AF (POAF) is a common complication in CAD patients undergoing coronary artery bypass grafting (CABG) surgery, and is associated with increased 10-year mortality risk.62 Preoperative estimation of the risk for POAF remains a great challenge.63 Circulating miRNAs have been proposed as potential biomarkers to predict the risk of POAF after CABG surgery (Table 3).
In 2017, Harling et al. found that increased preoperative serum levels of miR-483-5p could help to predict the risk of developing POAF in patients undergoing CABG revascularisation.64 They enrolled a prospective cohort of 34 UK patients undergoing non-emergent, on-pump CABG, including 13 who subsequently developed POAF (mean age 64.6 ± 11.3 years) and 21 without POAF (mean age 59.6 ± 12.1 years), matched for age, sex and TRFs, and without previous history of AF. Using a microarray approach, they first investigated the entire miRNAome in the atrial tissue, taken intraoperatively before bypass instigation, of a subgroup of 11 patients with POAF and 11 matched patients without POAF. Then, they quantified by RT-qPCR in the whole cohort the preoperative serum levels of the most upregulated and the most downregulated of the 16 differentially expressed miRNAs; that is, miR-483-5p and miR-208a, respectively. Interestingly, they observed significantly higher levels of miR-483-5p in preoperative serum of POAF patients. The authors reported a predictive ability of this miRNA, as indicated by a significant AUC.
Feldman et al. in 2017 investigated the potential of serum miR-1, miR-23a and miR-26a in identifying patients who develop PAOF after CABG.65 These miRNAs were selected based on previously reported associations with AF.66 Using RT-qPCR, they measured preoperative and postoperative (48 h) serum levels of the three miRNA in a cohort of 48 Brazilian patients undergoing CABG surgery, including 24 POAF (mean age 64.7 ± 7.5 years) and 24 non-POAF (mean age 56.3 ± 9.2 years) matched for sex and TRFs. They found no difference in preoperative circulating miRNA levels between the two groups. Conversely, they observed that miR-23a and miR-26a were significantly reduced in POAF patients after surgery, and showed a moderate predictive ability.
In 2020, Rizvi et al. proposed a preoperative multiparameter biomarker to identify the risk of new-onset POAF, which included serum levels of miR-29a.67 They hypothesised that combining clinical risk factors for AF with markers of the atrial pathophysiological substrate (fibrosis) and its regulatory miRNAs might have incremental value in identifying patients at risk of POAF. They measured preoperative serum concentrations of peptides reflecting collagen synthesis and degradation, extracellular matrix protein synthesis and deposition, and of miR-29s (miR-29a, miR-29b and miR-29c) known to modulate fibrosis, in a US cohort of 90 patients scheduled for an elective CABG procedure with no prior AF (34 with POAF, mean age 72.4 ± 10.8 years, and 56 without POAF, mean age 67.3 ± 10.7 years).68 They observed that procollagen III N-terminal and procollagen I C-terminal peptides were elevated in POAF compared with non-POAF patients, while miR-29 levels were reduced. These markers showed a correlation with atrial fibrosis extent. The model with the highest accuracy in identifying POAF patients was obtained by combining age with procollagen III N-terminal peptide and miR-29a, as assessed using the receiver operating characteristic curve by fitting logistic regression with POAF as the outcome.
Challenges of MicroRNAs as Prognostic Biomarkers
These studies highlighted the potential of circulating miRNAs as promising biomarkers with prognostic value for MACE, mortality or POAF in patients with CAD. Specifically, higher plasmatic levels of miR-208b and miR-499-5p were found to be associated with an increased risk of mortality in different studies on ACS cohorts (Table 2).47,50,51 However, there is little overlap in many experimental findings and there is no agreement in the studies on POAF. Surprisingly, in different studies, miR-19a, miR-133a and miR-223 showed opposite regulation in baseline samples of future cases in patients with ACS compared with those with chronic coronary syndrome.47,53,55,60 Intriguingly, miR-126-3p, miR-197 and miR-223 were identified as predictors of all-cause or cardiovascular death in both general population cohorts and cohorts of documented CAD patients, thus extending the applicability of these circulating miRNAs to primary and secondary prevention.26,32,53,57,60
The inconsistent results mentioned above are due to several factors. As with studies on the predictive role of miRNAs, the most critical issues are sampling, normalisation method and clinical endpoint. The clinical outcomes considered were diverse, including either all-cause or cardiovascular death, or a composite endpoint, such as MACE. Other factors influencing reproducibility could be the limited number of cases in many of these studies, the different composition and/or ethnicity of the populations examined, different methodological approaches and analytical strategies.
Limitations of MicroRNAs in Modern Cardiology Practice
Despite their potential, several problems need to be overcome before circulating miRNAs can be used in clinical practice. An important issue to be addressed is the implementation of standard operating procedures for sample preparation, miRNA isolation, quantification and normalisation across multiple samples, as the sensitivity of diverse methodologies could be substantially different and could lead to inconsistent results.46,69 Measuring circulating miRNAs is still challenging due to their low concentration, and a univocal consensus on miRNA quantification methods is still lacking. The main detection methods available are RT-qPCR, next-generation sequencing and microarrays. RT-qPCR is the most widely used method, but the various approaches can yield significantly different results: universal RT or RT with specific primers; preamplification; specific amplification primers with a DNA-binding dye (SYBR Green), or target-specific primers combined with dual-labelled probes that hybridise to complementary target sequences (TaqMan). Next-generation sequencing is highly processive, has no probe-related bias, and enables the discovery of novel miRNAs, but protocols are still cumbersome compared with the other two techniques. Finally, microarrays are relatively inexpensive probe-based assays that require a preamplification step and are less sensitive than the other two techniques.70
In addition to poorly defined laboratory standards, another crucial issue is the lack of sufficient evidence.
The data presented here underline the need for well-designed clinical studies in larger prospective cohorts of patients, using standardised protocols. Larger sample sizes are crucial to distinguish between healthy or diseased status, and dissect the contribution of confounders, such as age, sex, ethnicity, lifestyle, pre-treatment and disease history. Despite encouraging results, miRNAs are not currently used for the risk stratification or prognosis of CAD. Indeed, although circulating miRNAs have been shown to add predictive capacity to TRFs-based risk scores in several primary prevention studies, the poor reproducibility of the results and the different clinical endpoints considered limit their prospective use in clinical practice.26,29,31,32,43
Furthermore, single miRNAs are rarely disease-specific, whereas combining miRNAs in panels increases specificity and slightly/moderately increases the predictive power of conventional scores. In contrast, secondary prevention studies have also provided conflicting results. In two of them, the miRNAs identified in ACS patients did not add prognostic power to hsTnT.47,50 In other studies, single miRNAs or miRNA panels have shown prognostic ability independent of various TRFs and established biomarkers, but once again there was little consistency and different associations with mortality, positive in ACS patients and negative in patients with chronic CAD.53,55,60 In general, there is a lack of evidence that circulating miRNAs can increase the prognostic power of established biomarkers, such as LDL cholesterol, high sensitivity troponins and N-terminal prohormone of brain natriuretic peptide.
Conclusion
MiRNAs are highly investigated for their role in the pathogenesis of CAD. Nevertheless, evidence for clinical implementation is still lacking. The results of our review show that there is currently insufficient support for the use of any of the presented miRNAs as predictive or prognostic biomarkers in clinical settings. However, evidence highlights the potential of circulating miRNAs as novel biomarkers for both primary and secondary CAD prevention. Only a few miRNAs showed risk-predicting ability in more than one study, underscoring the need for further investigation in larger cohorts with standardised methodologies. In particular, standardising miRNA selection approaches, normalisation strategies, and adjustment for potential confounders will ensure improved reliability and reproducibility of results, and allow adequate comparison between studies. Once consensus is reached, miRNAs could be used for the development of novel risk stratification algorithms.